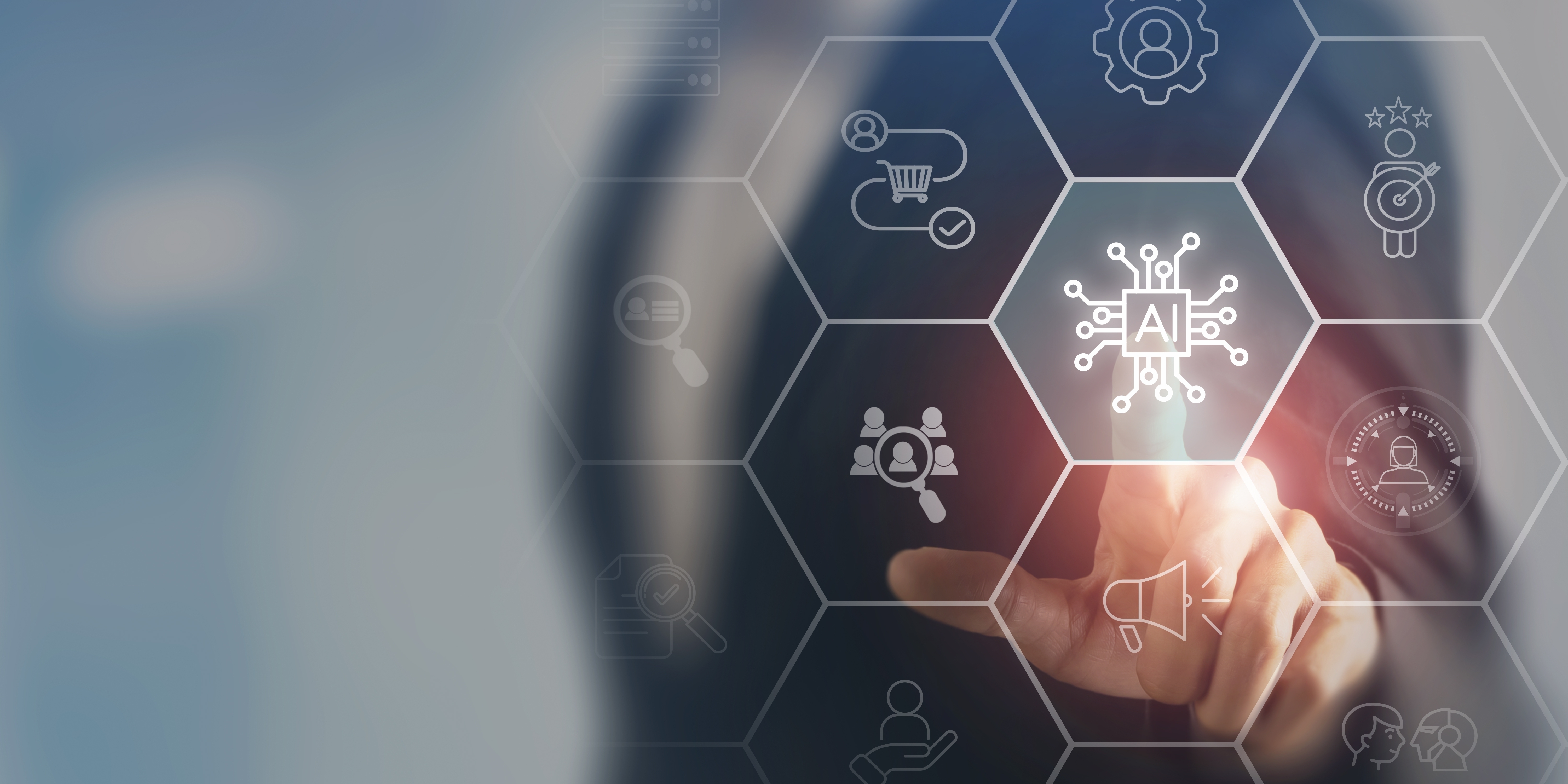
Introduction
We are living in an exciting time, right in the middle of a tech revolution. The ever changing technology is helping businesses solve innovative challenges, and at the same time, user expectations are changing faster than ever. To stay ahead, businesses must anticipate and adapt to these changes. Understanding and meeting these expectations is critical because how users perceive and interact with technology can significantly impact their trust and satisfaction.
The key to this transformation? Design for Intelligence—a shift from static experiences to intelligent ones where businesses integrate Artificial Intelligence (AI) and user-centric methodologies to create smarter, adaptive, and personalised experiences. It’s about crafting experiences that understand, learn, and adapt to user needs in real time. It also requires a proactive mindset where businesses embrace data-driven insights to craft these intuitive experiences.
Early adopters won’t just meet expectations—they’ll help set new industry standards. As the market increasingly demands immediacy, personalisation, and seamless integration across products and services, adopting design for intelligence becomes not just an opportunity but a necessity for long-term success.
The question is not if businesses should embrace this change, but how fast they can do so.
This will essentially drive businesses:
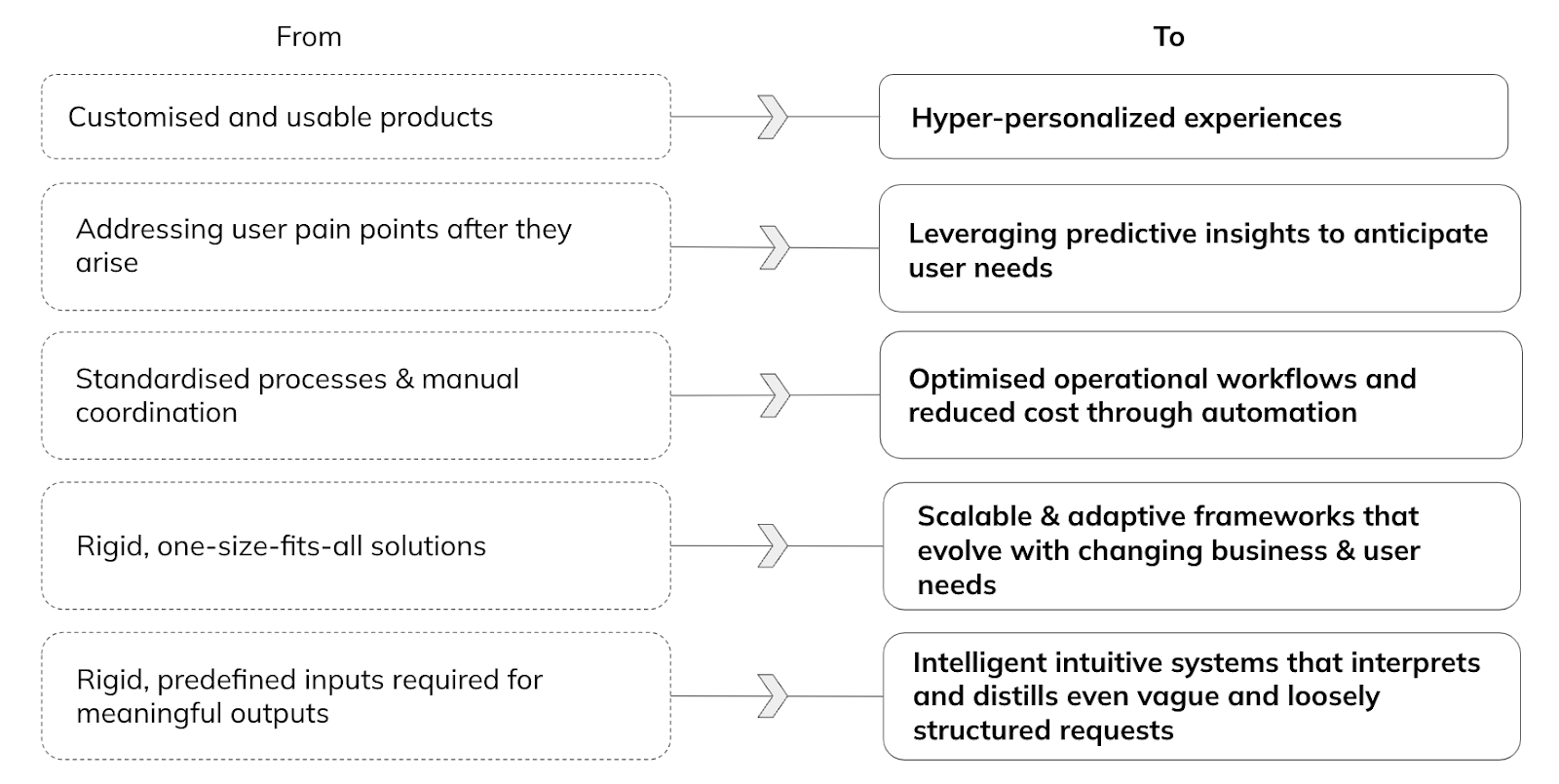
Let’s understand the above with the help a a banking application use-case.
Hyper-personalisation
A strategy that uses real-time data and AI to deliver highly tailored content and experiences to individual customers. It aims to treat each customer as an individual with unique preferences, thus enabling businesses to provide a unique experience for each user.
For example, instead of showing the same landing screen to all customers, the banking app can dynamically prioritise actions based on what the user frequently does (e.g bill payments, fund transfers, etc.)
Leveraging Predictive Insights
By using Machine Learning (ML) models built on real-time data and AI, businesses can anticipate and address user needs before they arise.
For example, if a customer’s travel spending increases, the app suggests a travel credit card with better rewards.
Operations Optimisation Through Automation
Traditional operations require a lot of manual checks, approvals and often have redundant processes. Based on customer data, systems can automate these processes and bring a lot more seamlessness and efficiency.
For example, offering instantly approved loans by assessing spending behaviour and financial health without needing manual checks.
Scalable and Adaptive Frameworks
As customer needs and wants evolve dynamically, businesses must adapt to continue delivering value. Using flexible workflows and technology, businesses will be able to adapt to evolving environments and expectations.
For example, developing dynamic risk assessment models that adapt to changing market conditions and customer behaviours.
Intelligent and Intuitive Systems
Traditional systems handle user requests by asking clarifying questions. For instance, if a user says, “Help me sort out my payments,” the system might respond with, “Do you mean bill payments or loan payments?”
In contrast, an intelligent, intuitive system analyses past transactions, detects overdue bills or recurring payments, and proactively suggests actions. This shift makes interactions seamless, intuitive, and proactive, significantly reducing user effort.
Creating Smarter Workflows for Banking Apps
Smarter workflows are no longer a luxury—they are a necessity. The era of one-size-fits-all banking experiences is over. Every user has unique financial needs, behaviours, and ways of interacting with their bank. With AI-driven intelligent workflows, banking apps can now move beyond static interfaces to dynamically adapt to individual user patterns.
![]() | The Current State of Banking AppsMost banking apps today follow a standard structure:
While this approach offers convenience, it doesn’t necessarily provide intelligence. Every user is treated the same, even though their banking habits vary significantly. |
Rethinking Banking Journeys with Customer DNA
With the vast amount of user data available, banks have an opportunity to go beyond generic workflows and create Customer DNA—a personalised understanding of each user’s financial behaviours and needs.
Customer DNA is built with three components:
Individual Insights: Tells about customers behaviour and patterns based on past usage
Journey Context: Understands customers environment and parameters that triggered usage
Situational Awareness: Tells about current state and external factors that can influence customer usage and decision making
By mapping this data and integrating AI-powered decision engines, banks can predict and preempt user needs rather than waiting for them to initiate transactions.
The Power of Predictive Workflows
![]() | Imagine logging into your banking app and instantly seeing: With predictive modelling and intelligent design, banking apps can anticipate user actions, reduce friction, and streamline workflows—making banking seamless, intuitive, and hyper-personalised. |
Turbocharging Smarter Workflows by Understanding Customer Journeys and Model Context Protocols
We spoke about how these seamlessly integrated, intelligent and context aware new workflows will help banking users. However, with the advent of Model Context Protocol (MCP), these workflows are no longer confined to standalone apps; they now extend into user’s real-world actions across platforms. This unlocks a whole new layer of frictionless finance.
MCP is an emerging standard that allows AI models to interact seamlessly with various applications and services outside their native platforms.
By understanding the customer journey and the context, banking apps can expose relevant workflows to AI models and can enable cross-platform intelligence and proactively assist users across platforms like e-commerce, hospitality, healthcare, et al.
For example: A banking app detects a flight booking and instantly provides travel insurance options or foreign currency recommendation without the user’s intervention or the bank detects a subscription renewal (Amazon Prime, Spotify) and prompts the user to confirm, cancel or postpone based on the spending patterns.
Moving from Traditional Banking to AI-driven Smart Workflows
While this sounds amazing, however, in order to move from the current state of static banking workflows to smarter and intelligent workflows will require a solid foundation of data engineering practices, ML models and adaptive user experience designs incorporating the feedback loop.
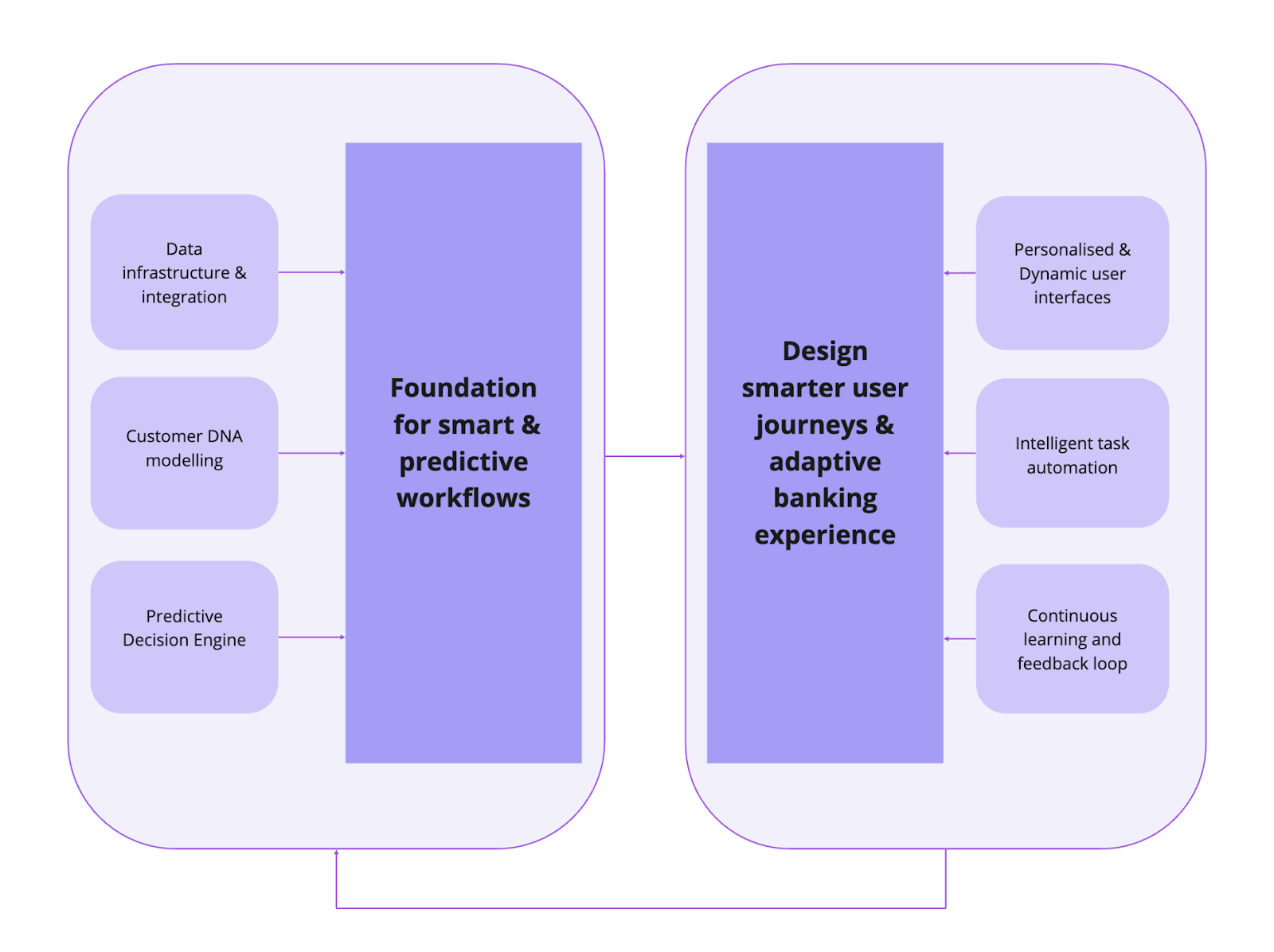
1. Building the foundation for smart and predictive workflows:
To transition from traditional banking experiences to smarter, predictive banking workflows, financial institutions need to focus on three key areas:
a) Data Infrastructure and Integration
A rich and actionable dataset (spanning both structured and unstructured sources) is going to be back-bone for this. While there is available user data, generating insights from user data and ensuring that it is high quality via data quality checks is going to be a crucial and important part of this process. Once this is done, will have to implement real-time data pipelines that will allow banks to update and create a holistic customer profile. And not to forget to ensure compliance with financial regulations (GDPR, banking regulations, et al).
b) Customer DNA Modeling
We looked at Customer DNA above and it will require understanding the user’s individual insights, journey context, and situational awareness. Essentially to move beyond basic demographics and segment customers dynamically based on behavioural patterns, lifecycle stages, and financial goals.
Machine learning models would help in analysing past interactions and transactions to predict the next best action.
c) Predictive Decision Engine
This AI-driven engine acts as the brain behind the personalised workflows.
For example in pattern recognition by identifying recurring financial actions and anticipating when they will be needed or by providing smarter nudges based on user behaviour and market trends.
2. Designing Smarter User Journeys
Once we have the Customer DNA and predictive intelligence in place, the next step is to redefine banking interactions by designing adaptive and intelligent user journeys.
These smarter journeys will help in moving away from static dashboards and will act as a layer on top of existing journeys. The aim is to shift to contextual interfaces where the application dynamically adjusts based on what the user is likely to do next.
At the same time, the models should evolve based on user behaviour shifts and user feedback to make the overall experience unique and trustworthy.
Thin Slice Approach with an Iterative Design Framework
Smarter and more intelligent workflows will be the key differentiators in the future of digital banking. However, transitioning from the current state to the north star of predictive workflows requires a strategic, iterative approach rather than an all-at-once transformation.
A thin slice approach focuses on incremental improvements by identifying high-impact use cases, user behaviours and patterns, and optimising workflows around them. By tackling one core experience at a time, banks can progressively build towards a fully intelligent ecosystem.
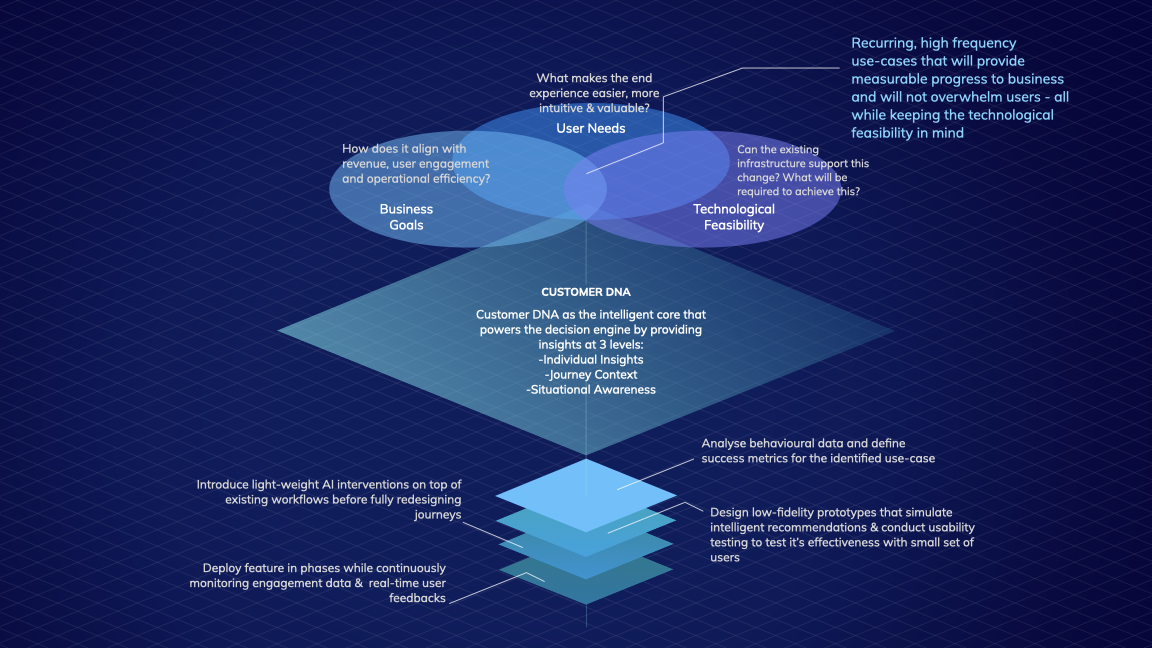
A high-frequency use case that aligns well with the business goal and technological feasibility could be setting up manual payments. Now when we are understanding the user pain-points and opportunities for automation, we also need to understand what does ‘smart’ mean to the user in this context – is it all about saving time, and reducing errors?
To illustrate the above using an example:
Let’s say the idea is to introduce an AI-powered prompt that suggests a one-tap payment for recurring bills. A prototype for the same could test whether users prefer a predictive payment suggestion vs. a manual transaction setup. Now, while implementing a layered approach, instead of replacing the traditional transaction page, ‘Smart Suggestions’ module can be introduced that learns from past behaviours. Finally, if users frequently dismiss a predictive transaction suggestion, analyse why – was it poor timing, incorrect data or simply lack of trust. Optimise it further based on the feedback and bring changes that help in adoption.
Key takeaways for iterative design approach:
Start small and validate early
Leverage AI as a guide, not a replacement
Design with transparency
Evolve through real-world data
In conclusion, data-driven, AI-enhanced experiences are the future. The real question is: Is your product ready for it?